How AI is Reshaping the Future of Demand Forecasting
- Team Aays
- Dec 2, 2024
- 9 min read
Updated: Dec 18, 2024
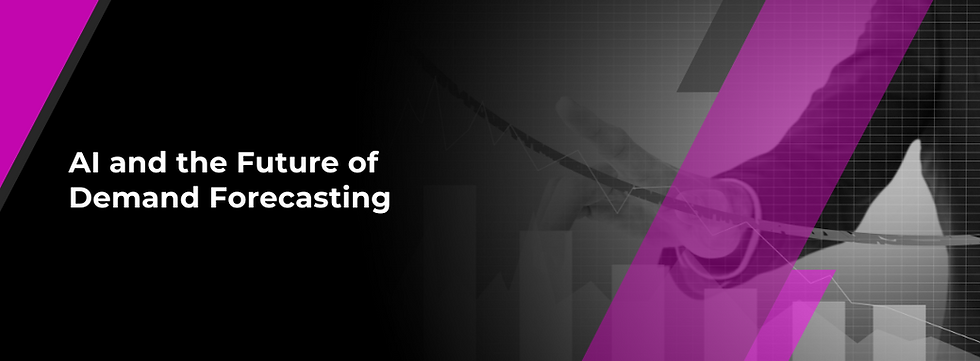
As organisations navigate increasingly dynamic markets, accurate demand forecasting has become critical for maintaining a competitive edge. AI-powered forecasting methods enable companies to analyse vast datasets, uncover hidden patterns, and respond to fluctuations with agility. This is particularly valuable for key stakeholders such as CFOs, COOs, and teams responsible for demand and supply planning, logistics, and inventory management. With AI driving efficiency, cost savings, and operational resilience, it empowers decision-makers to adopt smarter forecasting strategies and unlock significant value across the supply chain.
The Importance of Efficient Demand Forecasting
Efficient demand forecasting is indispensable for industries with high demand variability, such as retail, manufacturing, and supply chain. Accurate forecasting allows businesses to plan resource allocation, minimise stockouts, and reduce excess inventory, directly impacting cost savings and customer satisfaction. With AI-based demand forecasting, organisations achieve unparalleled efficiency, enabling them to adjust to market shifts in real time. For senior leaders, adopting AI-powered demand forecasting is not just about cost efficiency; it’s about building a proactive strategy to meet customer demands consistently and effectively.
Difference Between AI-Powered vs. Traditional Demand Forecasting
The shift from traditional to AI forecasting techniques brings significant improvements. Below are the strategic advantages of using AI for demand forecasting over conventional methods:
Comprehensive Data Integration: Using AI, businesses can process vast datasets by integrating historical and real-time data for robust analysis, unlike traditional models that primarily rely on past data.
Improved Forecast Accuracy: With AI-driven techniques, forecasting adapts to market changes, enhancing accuracy and reducing error rates.
Dynamic Adaptability: AI-based methods adjust to evolving demand patterns, addressing unexpected fluctuations more effectively than traditional approaches.
Faster Insights and Decisions: Leveraging AI allows businesses to gain rapid insights, enabling swift responses to changing market dynamics.
Reduced Manual Effort: By using AI, organisations can reduce the need for manual intervention, whereas traditional forecasting requires significant human input.
Enhanced Cost Efficiency: AI-driven forecasting optimises inventory and reduces wastage, driving cost efficiency across operations.
How AI Enhances Demand Forecasting
AI demand forecasting enables companies to process data in real-time, providing up-to-date insights and forecasting accuracy. This ability to analyse current data allows businesses to anticipate demand shifts, adapt strategies, and maintain optimal inventory levels. For decision-makers, AI-driven forecasting represents an upgrade in precision, supporting agile planning.
Key Benefits of AI in Demand Forecasting
AI in demand forecasting offers several strategic benefits, including:
Reduced Forecasting Errors: AI’s predictive accuracy minimises errors, improving decision-making.
Optimised Inventory Levels: AI helps align inventory with demand, lowering the risk of overstock and stockouts.
Cost Savings: Streamlined inventory and resource allocation reduce operational costs, enhancing profitability.
Enhanced Customer Satisfaction: Accurate forecasting ensures timely product availability, improving customer experience and loyalty.
AI Techniques Transforming Demand Forecasting
Several AI forecasting techniques help transform demand forecasting, including machine learning, neural networks, and predictive analytics. Each technique contributes unique strengths to forecasting accuracy, supporting senior leaders in aligning forecasts with market demands, optimising inventory, and enhancing resource management for sustainable growth.
Machine Learning Models for Forecasting
Machine learning models excel at recognising patterns in demand data, learning from historical trends and adapting to new inputs. These models can quickly identify seasonal trends and demand spikes, offering business leaders insights into customer buying patterns and market shifts. Machine learning’s adaptability in demand forecasting supports efficient operations and aligns resources with dynamic demand cycles.
Neural Networks in Forecasting
Neural networks, with their multi-layered structures, use advanced algorithms to analyse complex data patterns, making them highly effective for demand forecasting. Unlike traditional models, they capture subtle dependencies between variables—such as seasonal trends and economic shifts—that would otherwise go unnoticed. This capability allows leaders to optimise inventory, reduce costs, and improve resource allocation, resulting in more agile and cost-effective operations.
Predictive Analytics and Big Data
Combining predictive analytics with big data provides highly efficient AI forecasting. By processing large datasets across various sources—such as customer behaviour, market conditions, and seasonal fluctuations—predictive analytics helps businesses anticipate future demands, mitigate risks, and improve operational efficiency.
Streamlining Demand Forecasting Workflow with Generative AI
Generative AI transforms demand forecasting by delivering precise predictions, optimising workflows, and enabling faster data-driven decision-making. By processing large datasets and uncovering actionable insights, this technology supports efficient planning, resource optimisation, and adaptability to market changes, creating a competitive advantage.
Market Analysis: Advanced Insights for Strategic Planning
Generative AI enhances market analysis by identifying patterns and trends across vast datasets, enabling businesses to anticipate demand fluctuations and align strategies accordingly. Organisations can leverage these insights to adjust supply chains, production schedules, and marketing efforts in anticipation of changing market dynamics, ensuring they stay ahead of competitors.
Demand Planning: Precision in Uncertain Markets
Generative AI provides dynamic demand planning by analysing historical data, external variables, and real-time factors like weather or economic shifts. This adaptability ensures accurate demand projections, reducing risks like overproduction or stockouts. AI-driven demand planning also enhances operational flexibility, helping businesses respond quickly to evolving market conditions while minimising waste.
Inventory Management: Smarter Stock Control
AI-powered inventory management tools enable precise stock level optimisation, reducing overstock or shortages. By analysing demand patterns and replenishment needs, Generative AI ensures seamless supply chain operations and cost efficiency. Businesses can maintain consistent service levels, meeting customer expectations while optimising carrying costs and improving cash flow.
Resource Allocation: Efficiency in Operations
Generative AI forecasts resource needs, such as labour, materials, and equipment, with remarkable accuracy. This precision allows businesses to allocate resources effectively, reducing operational costs and improving productivity. AI-driven resource planning ensures organisations can scale operations efficiently during peak periods or adjust seamlessly to shifts in demand, supporting overall performance goals.
Key Steps for Implementing AI in Demand Forecasting
Implementing AI demand forecasting requires a structured, goal-oriented approach. Businesses should set clear objectives, prepare high-quality data, select suitable algorithms, and integrate AI within existing systems. For senior leaders, this organised process ensures reliable and adaptable forecasting, enhancing accuracy and supporting the organisation’s competitive and strategic objectives.
Defining Clear Objectives and Project Scope
Setting clear objectives aligns AI forecasting projects with business goals. Defining measurable KPIs provides leaders with benchmarks to assess success, helping to gauge the project’s impact on demand forecasting.
Data Collection and Preparation for AI Models
High-quality data is essential for accurate AI forecasting. Leaders should prioritise data cleaning and preprocessing, as prepared data enables models to achieve high accuracy and supports better demand predictions.
Choosing the Right AI Algorithms for Demand Forecasting
Selecting the right algorithms is crucial for accurate AI forecasting. Consulting industry experts ensure that algorithm choices align with business-specific needs, enhancing model performance and forecasting accuracy.
Enhancing Model Inputs Through Feature Engineering
Feature engineering improves model accuracy by selecting and refining data points most relevant to forecasting. For senior leaders, this boosts AI forecasting precision, supporting effective, data-driven strategic planning.
Training the AI Model for Accurate Forecasting
The model training phase includes initial training and iterative refinement, which is essential for reliable demand forecasting. Well-trained models empower leaders with insights that enhance decision-making and operational efficiency.
Integrating AI Models with Existing ERP Systems
Integrating AI models within existing systems enhances their utility. Ensuring compatibility, security, and cost-effectiveness is crucial, allowing senior leaders to manage implementation smoothly while achieving forecasting accuracy.
Implementing Real-Time Data Integration for Dynamic Forecasting
Real-time data integration ensures AI forecasting adapts quickly to market changes. This is particularly valuable for senior leaders, as it supports agile decision-making in response to shifting demand trends.
Validation and Testing for Model Reliability
Rigorous validation and testing establish model reliability before deployment, minimising errors. This is essential for building trust in AI’s predictive capabilities and aligning demand forecasts with strategic business goals.
Developing Strategies for Continuous Model Improvement
Continuous model monitoring and updating adapt AI forecasting to changing demand patterns. For leaders, this ensures sustained accuracy and responsiveness, keeping demand forecasting aligned with evolving business goals.
Use Cases of AI in Demand Forecasting for Different Industries
AI-driven demand forecasting transforms industries by delivering tailored, data-driven solutions that boost efficiency and competitiveness. For senior leaders in retail, supply chain, manufacturing, and automotive sectors, AI in forecasting offers enhanced demand planning, inventory management, and strategic decision-making capabilities, creating operational efficiencies that meet sector-specific demands.
Retail
In retail, AI forecasting optimises inventory management, minimising stockouts and enhancing customer satisfaction. Using AI, businesses can predict sales trends to ensure products are available to meet customer needs, reducing waste and improving stock turnover. Retail leaders benefit from data-driven insights that support agile decision-making, aligned product availability, and operational efficiency. By anticipating consumer demand patterns, AI enables retailers to maintain optimal stock levels, build stronger customer relationships, and enhance the overall shopping experience, ultimately supporting business growth.
Supply Chain and Logistics
For supply chains, AI forecasting improves inventory control, route planning, and demand alignment. Using AI, businesses gain real-time insights for resource allocation, reducing logistics costs and enhancing flexibility in response to demand shifts. Leaders benefit from AI’s adaptability, supporting efficient supply chain operations and resilience. AI-based forecasting helps logistics teams optimise routes, ensure timely deliveries, and manage stock levels effectively. This approach enhances responsiveness, meeting market demands while reducing costs and supporting robust supply chain management.
Manufacturing
In manufacturing, AI demand forecasting predicts production needs, optimising inventory and resource use. Accurate forecasts reduce waste, cut costs, and support sustainable production. By leveraging AI, businesses can align resources with production goals, adapting swiftly to demand fluctuations. AI improves production scheduling, inventory control, and waste management, enabling manufacturers to maintain agility and support sustainability by aligning production with customer demand.
Automotive
In the automotive industry, AI in demand forecasting aids sales prediction and production planning, supporting leaders in proactive decision-making. Insights derived from AI allow manufacturers to respond quickly to changes in consumer preferences and market conditions, aligning production with demand patterns. This enhances resource management, reduces excess stock, and improves inventory turnover. Automotive companies benefit from accurate, timely insights into demand trends, ensuring that production aligns with market dynamics, ultimately supporting continuous adaptation and efficiency in a highly competitive sector.
Challenges and Considerations for AI Demand Forecasting
Implementing AI in demand forecasting brings challenges, including data privacy, training complexity, and initial costs. Addressing these requires transparent practices, workforce training, and long-term ROI evaluation. Data privacy remains a key consideration as companies handle extensive customer and operational data. Leaders must also consider model training complexities, ensuring teams are equipped to manage AI forecasting tools effectively. The upfront investment costs necessitate a strategic approach, focusing on phased adoption to manage resources. Overcoming these challenges ensures businesses remain competitive and agile in their demand forecasting while fully capitalising on AI’s potential for efficiency.
Best Practices for Integrating AI in Demand Forecasting
Successful AI demand forecasting integration begins with pilot projects, high-quality data, and cross-functional collaboration. Pilot projects allow teams to refine approaches, addressing challenges before full-scale implementation. Quality data supports model accuracy, improving forecast precision, while cross-functional collaboration ensures cohesive implementation across departments. These practices lay the foundation for seamless, adaptable AI integration. Senior leaders gain the insights necessary for accurate demand forecasting, enhancing strategic planning, resource allocation, and operational agility across the organisation.
Driving Business Value with AI-Enhanced Demand Forecasting and Aays
Using AI, Aays delivers demand forecasting and risk resiliency solutions that empower businesses to navigate market complexities with adaptability and powerful data-driven insights. Aays helps companies structure compliant AI frameworks that align with strategic goals, ensuring reliable and scalable forecasting and risk management.
Aays provides senior leaders with a suite of advanced demand forecasting and risk resiliency tools, including:
Predictive and Causal Modeling for Demand
Using AI, Aays enables demand forecasting that dynamically adapts to changes, enhancing forecast reliability and optimising resource planning.
Trade and Supplier Risk Management Co-Pilot
Powered by GenAI, this tool helps businesses proactively manage supply chain disruptions by synthesising insights from millions of external data sources, including news, climate data, and geopolitical information. The Co-Pilot provides a comprehensive risk score at supplier and country levels, contextualising these risks with internal data to quantify exposure across geographies, business units, and brands. It also simulates "what-if" scenarios to provide sourcing strategy recommendations, helping businesses mitigate risks and maintain resilience.
Explainable AI for Transparency
With interpretability built into its solutions, Aays ensures transparency in forecasting and risk assessments, allowing leaders to make decisions based on clear, actionable insights.
By partnering with Aays, organisations gain resilient, accurate demand forecasting and risk management solutions, driving growth and sustainability.
Frequently Asked Questions
How big is the AI demand forecasting market?
The AI demand forecasting market is growing rapidly as companies across industries recognise AI’s potential to enhance demand planning. With applications in retail, logistics, and manufacturing, this market is expanding as organisations adopt AI for precise forecasting, reducing costs, and improving customer satisfaction through optimised resource management and agile planning.
Comments